Overview
What is Apache Hive?
Apache Hive is database/data warehouse software that supports data querying and analysis of large datasets stored in the Hadoop distributed file system (HDFS) and other compatible systems, and is distributed under an open source license.
With Apache Hive, you can enter the world of Big Data
Best Distributed Database in the market
Help your dev team !
Spectacular SQL-like interface for accessing Hadoop
This system makes active data of value.
Best query platform for ETL.
It is an advance to the ease of the processes
Capabilities of Apache Hive
Excellent bigdata warehouse solution
Our use …
very useful for OLTP
Apache Hive
Walk into the World of Big Data with Apache Hive
Reliable and Cheaper one stop Data warehouse solution
Big Data the SQL way
Apache Hive: Big data querying tool w/SQL interface, but slower, more costly computation
Awards
Products that are considered exceptional by their customers based on a variety of criteria win TrustRadius awards. Learn more about the types of TrustRadius awards to make the best purchase decision. More about TrustRadius Awards
Pricing
What is Apache Hive?
Apache Hive is database/data warehouse software that supports data querying and analysis of large datasets stored in the Hadoop distributed file system (HDFS) and other compatible systems, and is distributed under an open source license.
Entry-level set up fee?
- No setup fee
Offerings
- Free Trial
- Free/Freemium Version
- Premium Consulting/Integration Services
Would you like us to let the vendor know that you want pricing?
24 people also want pricing
Alternatives Pricing
What is ClicData?
ClicData is a 100% cloud-based business intelligence platform that allows users to connect, process, blend, visualize and share data from a single place. As an automated platform, users are able to rely on the latest version of company data, to ensure users make the right decisions. Hundreds of…
What is retailMetrix?
RetailMetrix is a data analytics platform for retailers with the mission of enabling retailers to get value from their data. RetailMatrix processes and stores sales, labor and customer data using data warehouse technologies. Its dashboards and reports allows team to find the data that matters to…
Product Demos
Apache Hive Hadoop Ecosystem - Big Data Analytics Tutorial by Mahesh Huddar
Connecting Microsoft Power BI to Apache Hive using Simba Hive ODBC driver
Discover HDP 2.1: Interactive SQL Query in Hadoop with Apache Hive
Product Details
- About
- Tech Details
- FAQs
What is Apache Hive?
Apache Hive Technical Details
Operating Systems | Unspecified |
---|---|
Mobile Application | No |
Frequently Asked Questions
Comparisons
Compare with
Reviews and Ratings
(97)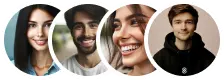
Community Insights
- Business Problems Solved
Apache Hive is a versatile software that has been widely used across various departments and organizations for different use cases. It has proven to be particularly helpful in handling large datasets, migrating data between different operating systems, synchronizing programs, and fetching and generating product metrics. Users have found value in using Hive for data analytics, engineering, data science, product management, and IT-related tasks such as improving analysis of big datasets stored in Hadoop HDFS.
Furthermore, Apache Hive has simplified the process of filtering and cleaning data using SQL, reducing the learning curve for handling big data. It allows users to run SQL queries against data in Hadoop, enabling efficient analysis of large datasets without the need to learn a new language. Additionally, Hive has been utilized for building reports, analyzing data stored in the Hadoop file system, processing events gathered in HDFS, and converting them into parquet files for fast querying.
Overall, users have praised Apache Hive for its scalability, accessibility, and cost-effectiveness in storing and retrieving analytics data. It has provided an intuitive solution for storing large datasets, querying big sets of data using SQL, aggregating massive datasets into distilled information for data-driven decision making, and creating external and internal tables in Hadoop/BigData projects. With its ability to process both unstructured and structured data efficiently, Hive has become an essential tool for data analysts, engineers, and business analysts across organizations.
Attribute Ratings
Reviews
(26-35 of 35)Apache Hive Review
- SQL like query engine, allows easy ramp up from a standard RDBMS
- Scalability is great
- If properly configured the data retreival is fantastic
- The way we currently have it implemented is quite slow, but I believe that's more of our implementation
- Joins tend to be slow
Apache Hive for ETL workloads
- Hive is good for ETL workloads on Hadoop.
- HiveQL translates SQL like queries into map reduce jobs.It supports custom map reduce scripts to plugged in.
- Hive has two kinds of tables- Hive managed tables and external tables.
- Use external table when other applications like pig, sqoop or mapareduce also using the file in hdfs. Once we delete the external table from Hive, it just deletes the metadata from Hive and original file in hdfs stays.
- Use Hive for analytical work loads. Write once and read many scenarios. Do not prefer updates and deletes.
- Behind scenes Hive creates map reduce jobs. Hive performance is slow compared to Apache Spark.
- Map reduce writes the intermediate outputs to dial whereas Spark operates in in-memory and uses DAG.
Apache Hive - Querying Big Data Made Easy!
Apache Hive solves a few issues for us but the main one being the ability to analyze large volumes of data on S3 directly with overall strong performance. We have been able to analyze billions of records in a matter of minutes with relatively small EC2 cluster using Apache Hive. It also allows for our Data Analysts to simply write SQL and avoids the ramp up to use other tools such as Apache Pig.
- Apache Hive allows use to write expressive solutions to complex problems thanks to its SQL-like syntax.
- Relatively easy to set up and start using.
- Very little ramp-up to start using the actual product, documentation is very thorough, there is an active community, and the code base is constantly being improved.
- Debugging can be messy with ambiguous return codes and large jobs can fail without much explanation as to why.
- Hive is only SQL-like, while more features are being added we have found that some things do not translate over (for example outer joins, inserts, columns can only be referenced once in a select, etc.).
- For out ETL jobs it does not seem to be the optimal tool due to tunings and performance being difficult, Apache Pig may be better for heavy processing jobs.
Hive Away, but not for everything!
- Hive which leverages traditional MapReduce at the core, can be used to process a large amount of data without a problem. Any problem that can be solved with MapReduce can now be simply expressed in SQL.
- Hive leverages the disk in the case of processing large data and is not limited by physical memory of any one machine (which is a limitation for systems like Presto). Hence it even allows reasonable fact-fact cross joins.
- Hive is extensible with UDFs. For any common patterns you can quickly write your own function set and it can be leveraged by everyone.
- SQL syntax of hive is unique and does not conform to ANSI SQL. This is quite painful for beginners.
- The ability to upsert records would be nice to have. Hive is cumbersome for mutable data where partitions require them to be rewritten. No one has solved this really well. If this is solved - it could be leveraged by many systems.
Easy access to data in Hadoop
- Faster than writing MapReduce or scalding jobs to access data in Hadoop.
- Syntax is essentially the same as that of SQL, making the barriers for entry to start using data low.
- Apache Hive can be quite slow and is not suitable for interactive querying. Simple queries will take many minutes and more complex queries can take a very long time to finish running.
Hive, last generation tooling but revolutionary for it's time.
- Connect BI tools to non relational data stores
- Simplify writing legacy MapReduce
- Speed needs to be a lot better
- Concurrency is not up to snuff
As sweet as Honey - Apache Hive
- Apache Hive works extremely well with large data sets. Analysis over a large data set (Example: 1PB of data) is made easy with hive.
- User-defined functions gives flexibility to users to define operations that are used frequently as functions.
- String functions that are available in hive has been extensively used for analysis.
- Joins (especially left join and right join) are very complex, space consuming and time consuming. Improvement in this area would be of great help!
- Having more descriptive errors help in resolving issues that arise when configuring and running Apache Hive.
Latency that exists when working with small data sets is a situation that needs to be looked at. Apache Hive is less appropriate in that scenario.
Hive brings the power of SQL to Hadoop
- Supports SQL like queries
- Various storage types including RCFile, HBase, ORC, etc.
- Supports indexing for acceleration
- HiveQL does not have all the features of SQL
- No support for transactions
HiveQL, Almost SQL, but not quite.
- Run SQL queries to an Hadoop cluster.
- Many different consoles can use it.
- Users don't have to write map reduce.
- Hive needs more SQL support.
- Enabling more date functions.
- Enabling more SQL table functions, such as inserting into a temp table.
Hive, a very powerful open source data warehouse solution.
- Partition to increase query efficiency.
- Serde to support different data storage format.
- Integrate well with Impala and data can be queried by Impala.
- Support of parquet compression format
- Speed is slower compared to Impala since it uses map reduce