Business Intelligence (BI) Tools
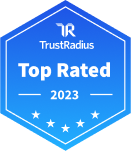
Top Rated Products
(1-5 of 8)
All Products
(1-25 of 564)
Explore recently added products
Videos for Business Intelligence (BI) Tools
Business Intelligence (BI) Tools TrustMap

TrustMaps are two-dimensional charts that compare products based on trScore and research frequency by prospective buyers. Products must have 10 or more ratings to appear on this TrustMap.
Learn More About Business Intelligence (BI) Tools
What is Business Intelligence (BI)?
Business Intelligence tools are designed to make sense of the huge quantities of data that organizations accumulate over time. BI tools analyze this information and present it as actionable information that can guide decision making.
Business Intelligence software (BI) makes up a large heterogeneous category of software. Not all tools in the category can be meaningfully compared to each other. There are several types of BI tools of which the most substantive are Full-Stack Business Intelligence Tools and Data Visualization Tools.
Business Intelligence Tools Features & Capabilities
- BI Platform Type
- Supported Data Sources
- Standard Reporting
- Ad-Hoc Reporting
- Report Output and Scheduling
- Data Discovery and Visualization
- Access Control and Security
- Mobile Capabilities
- APIs / Embedding
Full-Stack Business Intelligence Tools
Full-stack or traditional products are enterprise-level BI tools designed to solve the specific problem of enterprise data silos. Much crucial business data is stored in a range of different data stores, many attached to business applications like ERP. Full-stack BI tools consolidate all data in a data warehouse which is a relational database designed for data mining instead of transactional processing.
Slices of data from the warehouse—usually summary data for a single department like sales or finance—are stored in a “data mart” for quick access. All of this structured data is normalized by using an ETL process (Extract, Transform and Load) whereby transactional data is combined into a single pool of data and then “transformed” into a more appropriate format for querying and analysis before being written in the data warehouse database.
The Full-Stack Business Intelligence TrustMap shows only those products that belong in this sub-category.
Data Discovery and Visualization Business Intelligence
Data Visualization products have a different purpose. These BI tools are primarily designed to help data analysts discover patterns in large quantities of data and to build compelling visual representations which allow these patterns to be easily understood.
These business intelligence tools often use a technology called in-memory analytics to query data residing on a computer’s RAM rather than on a physical disk. This means that the data does not have to be stored anywhere for subsequent analysis.
Data visualization products often have lower cost of implementation and require less support. This can make them good business intelligence tools for small companies that don’t need a full-stack business intelligence solution.
The Data Visualization TrustMap shows only those tools that belong in this sub-category.
Business Intelligence and Reporting
Many BI tools also enable some forms of reporting. Modern BI’s ability to intake many different forms of data and present them in increasingly high and professional quality, some organizations are able to use their BI tool both to surface specific insights and generate regular reports. The level of automation found in BI reporting will vary highly from product to product. For general reporting needs, the ability to set-and-forget data pipelines into a BI tool and the normalization of functions like dynamic dashboards make some leading BI tools competitive automated reporting tools as well. However, specialized reporting cases that use more niche data structures may be less automatable in traditional BI tools.
Business Intelligence Software Comparison
When considering different business intelligence tools, consider the following aspects of each product offering.
- Ease of Use: Some BI tools offer high complexity and customization, but can be difficult for non-technical users. You should consider the technical ability of the staff that will be using the BI tools as well as if you need more complex features.
- Compatibility: Many BI tools are not complete, end-to-end solutions. For some organizations, purchasing BI software from multiple vendors may be necessary. If your business needs BI features that aren’t all covered in a single product, be sure to ask venders about integrations and compatibility with other software.
- Scalability: Some BI software doesn’t scale up very well as data needs increase. Consider the breadth of data generated by your organization and decide if that amount will stay the same or increase. If your data needs will increase, be sure to choose a BI solution that can handle your expected growth.
Start a Business Intelligence Software Comparison
Pricing Information
Business Intelligence software is generally quite expensive. However, it’s worth remembering that it is often money very well-spent in terms of significantly improved decision-making.
One of the impediments to understanding pricing is that vendors base their pricing on a broad range of different variables from number of users to number of CPU cores.
However, as a very rough guide, advanced report authoring can cost $2,000 to $2,500 per seat, while interactive report viewing can be about half that amount. Static report viewing can be as little as $50 per seat.